Good health is foundational to a fulfilling life. However, getting into a good state of health requires consistent effort, access to reliable information, and personalized guidance. Considering physician shortages, rising costs of care, and the blanket approach to treatment, meeting those basic requirements for good health remains difficult to achieve in practice.
Conversational AI in healthcare has become a vital part of the healthcare system that not only bridges the gaps in patient-provider communication but also gives patients more autonomy, control, and confidence in managing their own well-being.
What is conversational AI in healthcare?
Conversational AI in healthcare is an advanced AI technology that leverages advanced natural language processing, dialogue management, voice recognition, and other technologies to engage in dynamic and natural interactions with patients and aid healthcare professionals with their tasks.
Thanks to a complex combination of advanced technologies, conversational AI can operate in complex scenarios and provide a digital equivalent of bedside manner, drawing upon context, emotions, and patient concerns.
Conversational AI vs rule-based systems vs traditional machine learning chatbots
Below, our development team has curated the main differences between the three generations of chatbots to demonstrate what exactly conversational AI brings to the healthcare landscape.
Criterion | Rule-based systems | Traditional ML chatbots | Conversational AI systems |
---|---|---|---|
Underlying technology | Predefined logic trees and scripts | Conventional ML (e.g., decision trees, logistic regression) | A combination of advanced AI (natural language processing, dialogue management, LLM, speech recognition, etc) |
The depth of understanding | Basic keyword matching | Pattern-based understanding, limited natural language understanding | Advanced natural language understanding — understands intent, context, and sentiment |
Modalities | Text | Text and limited voice | Text, voice, and multimodal (text + voice + images + documents) |
Conversation flow | Static and linear | Semi-dynamic — the chatbot can handle simple multi-turns | Dynamic — the chatbot can handle multiple commands in a single utterance and multitopic conversations |
Context handling | None | The chatbot has short-term memory | The chatbot retains the memory across sessions |
Personalization capabilities | Unified experience for all users | Manual feature engineering allows for some personalization | Highly personalized, contextual experiences that adapt in real time to the user’s intent, tone, and profile |
Response generation | Predefined replies | Template-based replies | Human-like, contextually rich replies |
Adaptability | Manually updated | Requires retraining to evolve | Autonomously learns from new data, can adapt quickly with fine-tuning or new prompts |
When evaluating the type of chatbot you need for a specific healthcare task, you need to understand:
- What level of intelligence and nuance do you need?
- What modality does it have to operate in (text, voice, etc)?
- How much personalization and conversational dynamics does this use case need?
- How often does the knowledge base evolve?
Types of conversational AI tools, by the primary interface
Although the lines between the types of conversational AI solutions in healthcare have been blurring lately, there are three distinctive categories of this technology, each designed for a specific set of use cases.
AI-based chatbots
Primarily text-based, conversational AI chatbots live on hospital websites, healthcare apps, and patient portals. These solutions are designed to interact with users in real time, leveraging NLP to interpret user queries and deliver contextually relevant responses.
💡 Common use cases: symptom checking, triaging, pre-appointment procedures, and intake information collection.
Virtual assistants
Designed for hands-free, human-like interactions, virtual assistants typically belong to smart devices, ambient AI environments, and mobile health apps. They use voice interfaces to provide verbal responses to the user’s questions, perform tasks like appointment scheduling, and provide proactive support like medication reminders.
💡 Common use cases: medication management, patient engagement beyond hospital settings, elderly care, chronic care management, and daily health check-ins.
Interactive Voice Recognition (IVR) systems
The foundation of many healthcare call centers, conversational IVR systems leverage touch-tone input and the combination of advanced voice recognition and NLU to verbally interact with callers in a more empathetic way. Thanks to conversational AI, IVRs can build on the caller’s intent and emotional cues to provide more nuanced assistance, suggest more relevant options, and guide them through workflows in a more dynamic manner.
💡 Common use cases: appointment scheduling, insurance verification, prescription refills, test result inquiries.
Key applications of conversational AI in healthcare
Over 85% of healthcare providers in the U.S. have adopted AI-driven tools to elevate the patient experience and automate administrative tasks. Conversational AI is a core part of this adoption wave, bringing forth a versatile toolkit to address a multitude of healthcare needs.
Patient engagement and education
For any treatment to be effective, a patient needs to keenly understand their health condition, medical recommendations, and post-visit instructions. However, when leaving the appointment, patients tend to immediately forget 40% to 80% of the medical recommendations they receive.
Conversational AI solutions can pick up from where human doctors left off, providing patients with the necessary support and guidance post-visit or post-discharge:
- Reiterating the doctor’s post-visit/post-discharge instructions and summarizing them.
- Answering follow-up questions about medication dosages, activity restrictions, and more.
- Sending reminders for medications, follow-up appointments, and prescribed activities.
- Providing personalized condition-specific guidance to boost engagement.
- Tracking the patient’s progress and reporting potential issues to the healthcare team.
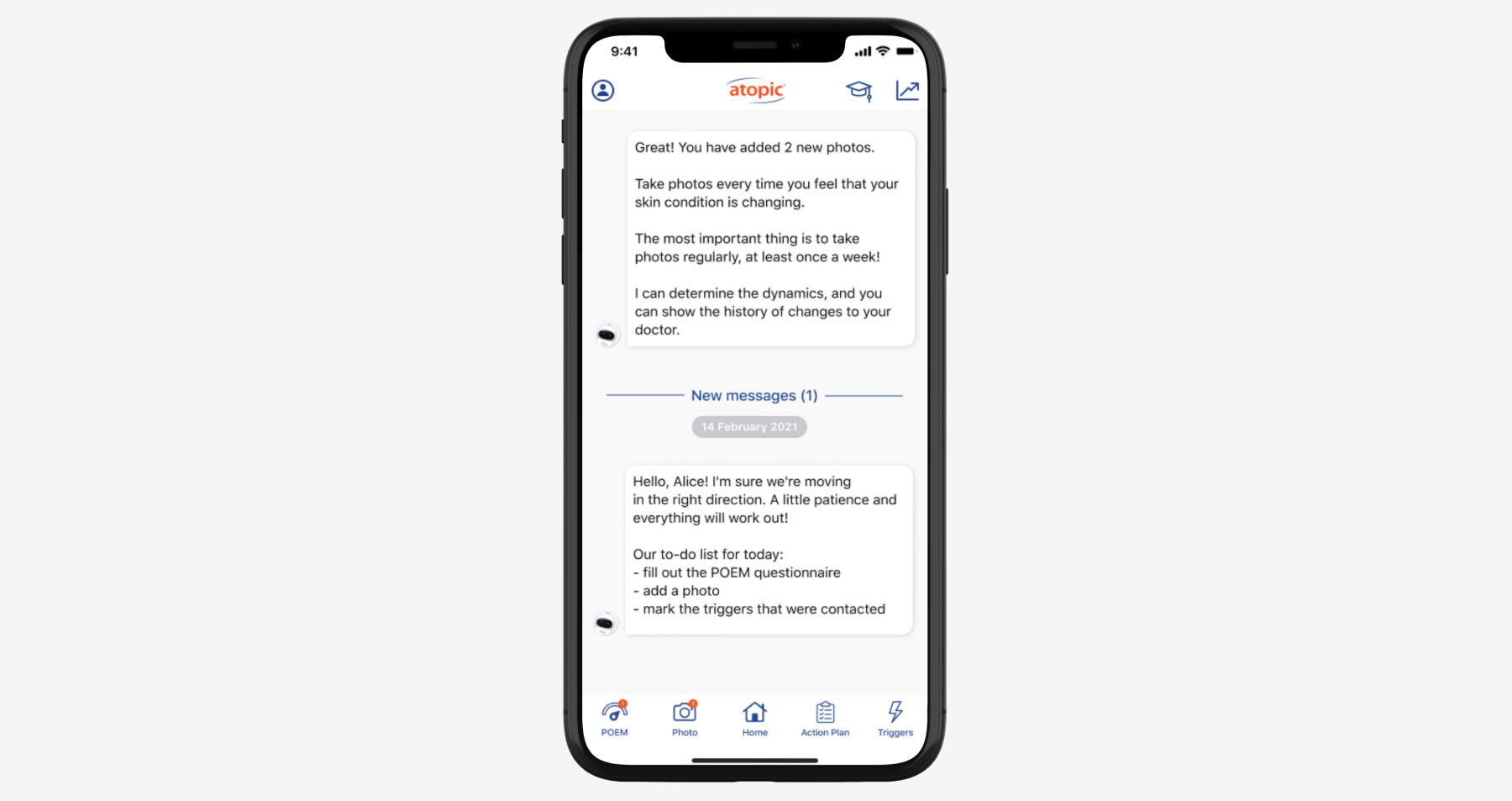
Administrative task automation
Сlinicians dedicate about 28 hours per week to administrative tasks while medical office staff set aside around 34 hours per week for similar duties. Integrating conversational AI into administrative workflows allows healthcare providers to reclaim their time and automate routine tasks with artificial intelligence.
Here are some administrative tasks in healthcare that lend themselves well to conversational AI automation:
- Collecting preliminary patient information before appointments (e.g., demographics, insurance details, current medications).
- Collecting initial information for referrals.
- Scheduling and managing appointments (maximizing visit blocks, guiding patients through scheduling, sending reminders, etc.)
- Triaging non-urgent patient messages and routing them.
- Handling follow-up care and scheduling additional procedures such as lab tests and imaging.
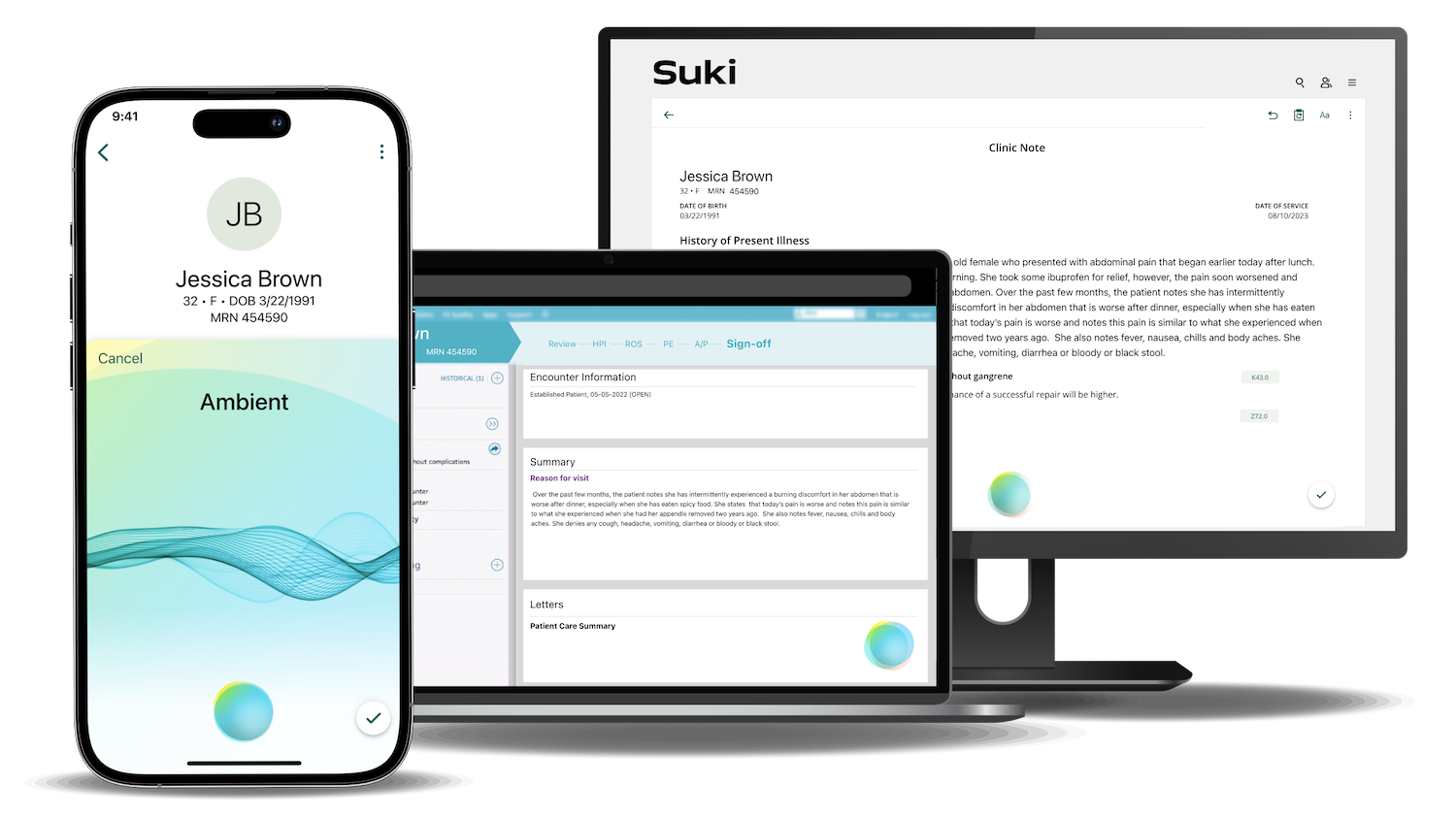
Clinical decision support
The road to an accurate diagnosis is a long one, not to mention expensive. Some conditions don’t fit into a neat little box, and others present with different symptoms across patients, throwing clinicians for a loop.
Linked to medical databases and clinical guidelines and fine-tuned with a combination of real-world and simulated medical dialogues, conversational AI healthcare systems can enhance physician decision-making to save time and save lives:
- Mapping symptoms to potential conditions and generating a differential diagnosis while accounting for patient complexity and patient context.
- Suggesting evidence-based diagnostic tests based on the patient's input.
- Flagging risk factors in real time based on the patient-reported symptoms.
- Summarizing clinical responses backed by citations.
- Coordinating and explaining medical image analysis, generating structured reports for radiology flows.
- Generating personalized treatment plans driven by the patient's medical history, genetic profile, and current condition.
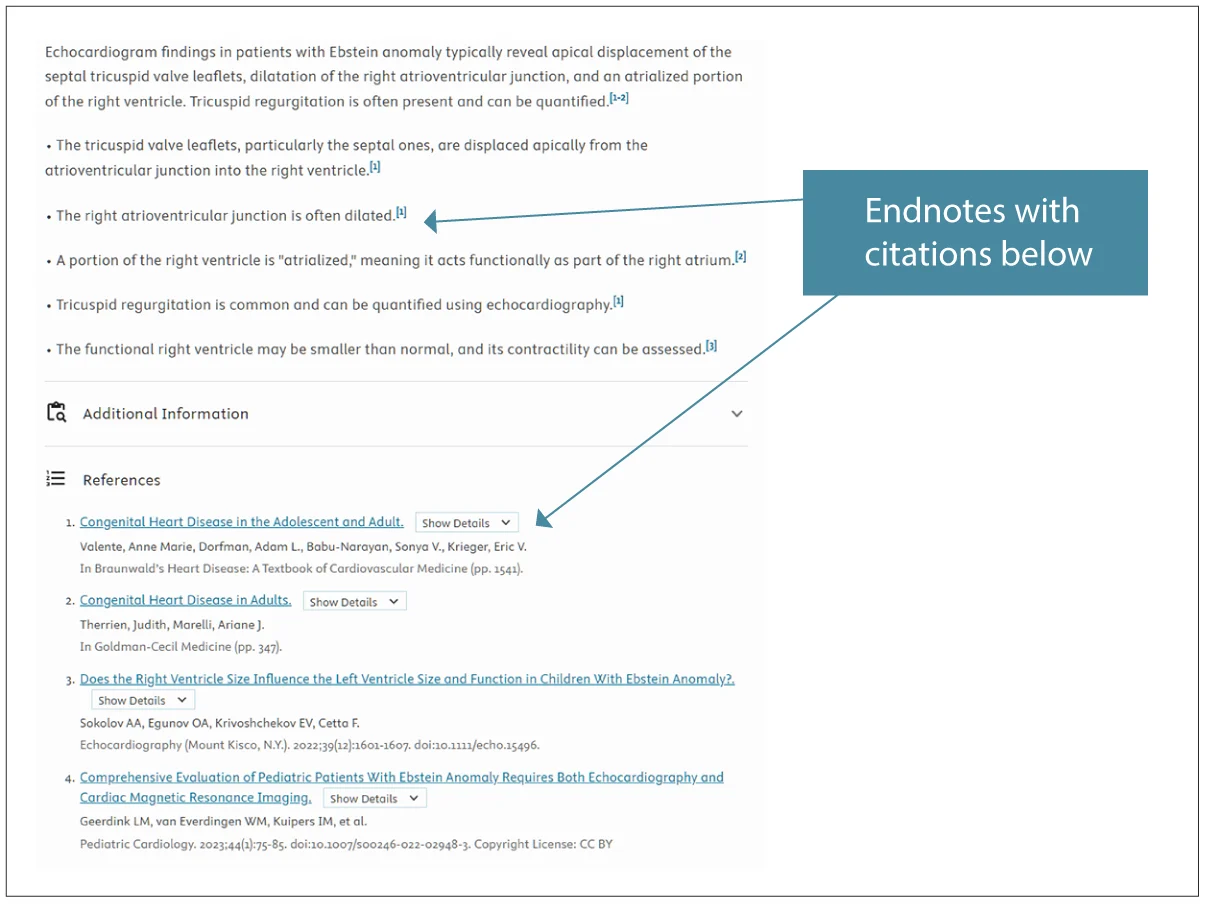
Keep in mind that conversational AI clinical decision support systems do not replace doctors and require doctor-in-the-loop mechanisms in place to keep human expertise central to the diagnosis.
Telehealth and virtual care coordination
In 2024, over 116 million users visited online doctor consultations worldwide, up from around 57 million in 2019. With the growing popularity of non-traditional care models comes the inherent complexity of coordinating care across providers, transitioning patients through various stages of virtual care, and supporting a reliable information flow between all sides of care.
Conversational AI for healthcare can streamline all those processes, improving health outcomes and patient satisfaction from afar:
- Before a visit, conversational AI interfaces can assist patients with intake, triage, and patient scheduling flows and check them for eligibility.
- During a visit, healthcare providers can rely on conversational AI tools for automated scribing, clinical support, real-time translation, and accessibility support.
- After a visit, artificial intelligence can generate follow-up instructions and reinforce patient education with personalized clinical and lifestyle guidance.
Also, conversational AI keeps everyone on the same page, gathering referral information, ensuring information transfer between offices, and automating outreach to patients.
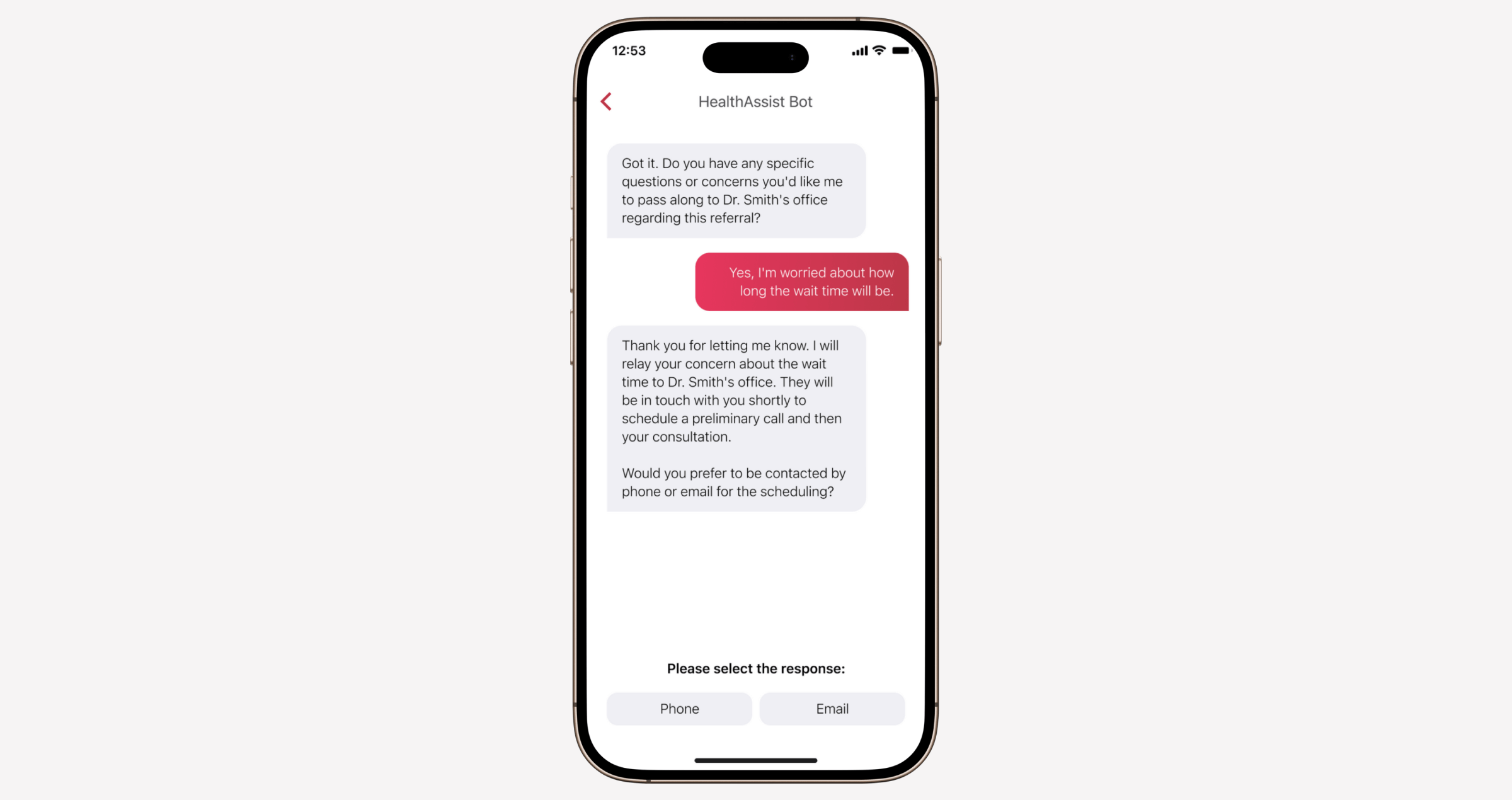
Medication management and adherence support
A lot of patients have complicated medication regimens, non-adherence, or mismanagement of which can lead to health deterioration and poorer patient outcomes overall. Available 24/7, conversational AI chatbots can monitor patients' medication adherence, helping them manage polypharmacy and deprescribing.
- Sending personalized medication reminders via text, voice calls, or in-app notifications.
- Tracking medication refills and proactively notifying patients of upcoming refill dates.
- Helping individuals create and maintain a list of all their medications, including prescribed ones, over-the-counter drugs, and supplements.
- Tracking missed doses in integration with smart pill dispensers.
- Identifying candidates for deprescription, generating a personalized tapering schedule, checking in for withdrawal symptoms, and more.
Mental health support and chronic disease management
Due to their evolving nature, mental health and chronic conditions require regular medical attention and active effort from the patient. With the specialist shortage creating a squeeze in access, healthcare conversational AI tools offer stand-ins for managing chronic conditions and mental health services.
As health management companions, conversational AI can both serve as self-care tools for patients and clinical decision support for healthcare organizations:
- Providing behavioral coaching and lifestyle support backed by evidence-based techniques.
- Gathering patient-reported data for the clinician’s review and treatment adjustments.
- Checking in with patients to record physical and mental health symptoms and notifying providers about potential complications.
- Offering emotional support and personalized coping strategies to individuals with mental health conditions.
- Preventing relapses by identifying changes in language patterns, engagement levels, emotions, tone, and more.
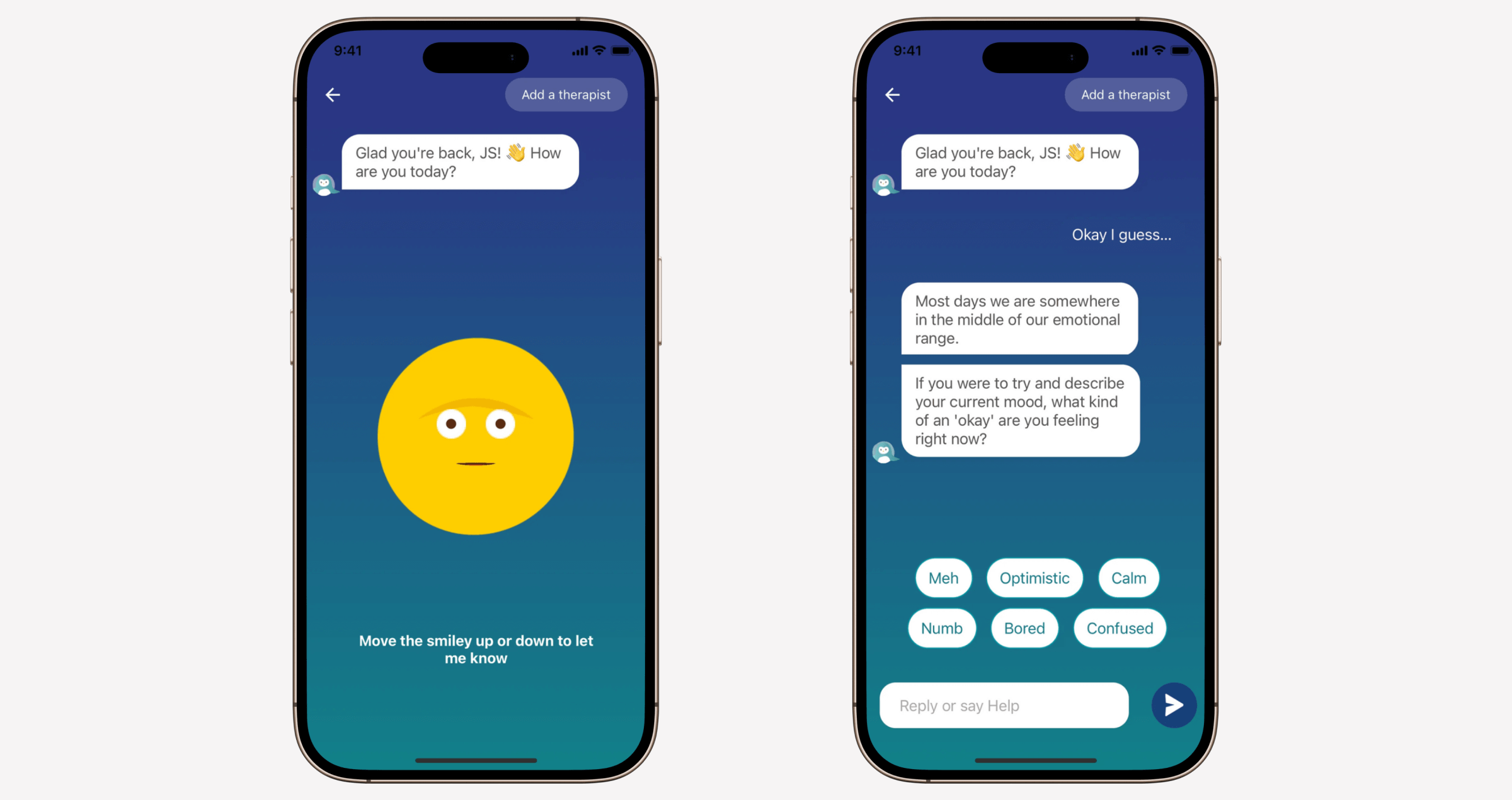
Pharmaceutical research
In the life sciences sector, the drug development phase alone can take 10 to 15 years, costing hundreds of millions to billions of dollars. Conversational AI systems can accelerate this process, automating and streamlining data-intensive processes across clinical trials and beyond.
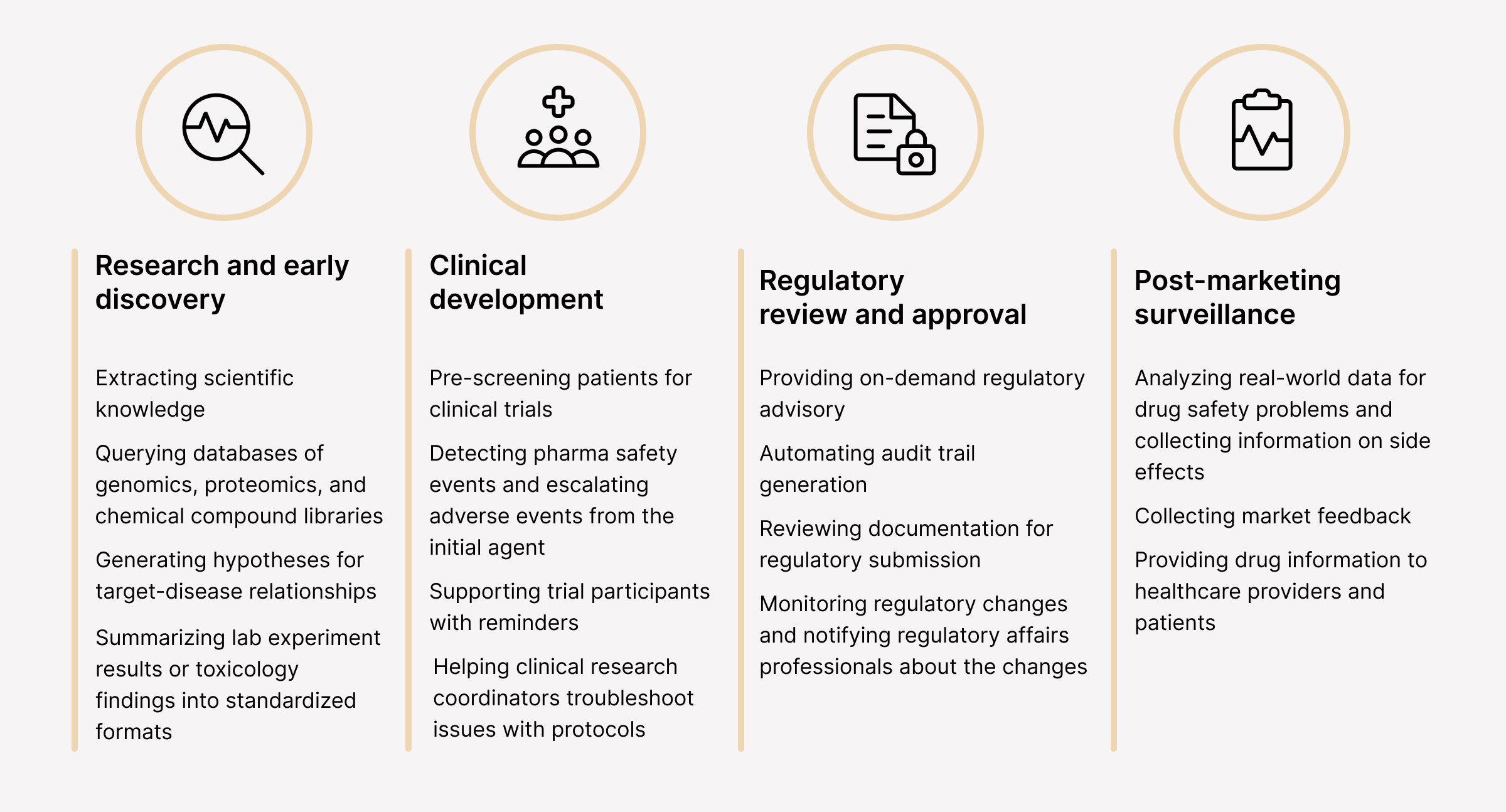
Insurance claims and billing assistance
In the healthcare sector, insurance claim denials are a widespread problem that can result in surprise out-of-pocket patient payments and disrupted revenue for healthcare providers.
By sharing this load with conversational AI systems in the following areas, healthcare providers can fast-track claims processing and slash the rate of denials:
- Mapping clinical language — Trained with clinical documentation and coding standards, conversational AI can do the heavy lifting of ICD-10 and HCC coding.
- Automating claims submission — Automatically populating claims forms based on the data from patient records and clinical notes.
- Verifying coverage — Conversational AI interfaces can run real-time eligibility checks to confirm deductibles and co-pays.
- Claim status tracking — Healthcare providers can use conversational AI to track claim status, while patients can turn to such interfaces for billing questions.
When wrapped into conversational AI, insurance cost estimator tools can also provide individuals with an estimate of potential out-of-pocket costs, taking into account the context, like the reason for a visit, to provide an accurate estimate.
Benefits of conversational AI for the healthcare industry
According to Hyro’s report, over 80% of consumers have used an AI chatbot or voice assistant in the past year for healthcare support. This growing adoption parallels the consumers’ need for faster, more accessible, and more personalized patient care. With conversational AI, healthcare providers can better meet those evolving needs.
Reduced administrative burden and operational costs
As a prescription for improved operational efficiency, conversational AI relieves healthcare staff of repetitive administrative burdens, including documentation, basic patient inquiries, appointment scheduling, and more. This saved time not only translates into better care but also equates to operational cost reductions thanks to the automation of previously manual tasks.
For example, 78% of physicians who use AI ambient listening reported it to accelerate clinical notetaking, with two-thirds noticing it also unlocks more time to spend more time on direct patient care.
Enhanced patient experience and satisfaction
Usually, doctors have around 15 minutes for each patient, which is barely enough for high-quality care, not to mention the requisite documentation. By automating in-visit documentation, conversational AI tools maximize the face-to-face time between the doctor and the patient.
Faster diagnoses, more personalized care plans, and proactive health interventions are all improvements that also became attainable thanks to AI's ability to analyze healthcare data. Between visits, conversational AI interfaces can guide patients through post-discharge instructions and medication management protocols, support remote healthcare delivery, and help with symptom self-management.
Better resource allocation
From patient triage to automated follow-ups and query routing, conversational AI allows healthcare professionals to focus on high-priority cases requiring immediate attention. Paired with automation and smart alerting, the technology allows more efficient use of time for nurses, physicians, and administrative staff.
Improved access to care
Thanks to 24/7 availability, patient-facing conversational AI platforms and applications can serve populations regardless of their location. Those with busy work schedules and limited transportation options can access healthcare services at their convenience and pace, wherever they are.
This flexibility, paired with the asynchronous nature of remote care tools, makes care more accessible and equitable, empowering individuals in rural and underserved communities with timely and convenient healthcare support.
How to implement a conversational AI solution in healthcare
Only 30% of AI pilots make it to production, with the rest being stalled by security, data readiness, integration costs, and other roadblocks. To turn your conversational AI experiments into real-world healthcare solutions with tangible results, you need a strategic approach to development.
Conducting a needs assessment
The development process unfolds with preliminary strategic planning that shapes the trajectory for your conversational AI software. At this stage, your development partner supports you in these key areas:
- Running a feasibility analysis to identify the requirements for your conversational AI business case.
- Determining behavioral and health outcomes you want to achieve with the conversational AI and planning the core functionality.
- Mapping your clinical pathways to identify the necessary integrations and data flows.
- Identifying where the solution stands in terms of regulations and planning the necessary safeguards to meet the regulatory requirements.
- Defining target users and performing design research to understand their needs, potential barriers to adoption, and more.
Planning the project and choosing a tech stack
Depending on the use case and modality of your conversational AI solution, the development team identifies the right set of technologies:
- Selecting the right tech stack and architecture, factoring in the scalability, security, interoperability, and generalizability needs.
- Laying down the security and compliance infrastructure (data encryption, privacy-by-design, etc.)
- Selecting suitable modality-specific technologies (NLP, NLU, speech recognition, etc.)
- Documenting architectural decisions, tech choices, security protocols, and integration strategies.
- Drawing up a detailed project development roadmap.
Preparing the data
Data preparation is a crucial stage of the conversational AI development process that directly impacts the success of your AI project. Here, the milestones include:
- Selecting relevant healthcare databases, along with the types of necessary training data.
- Cleaning, validating, and standardizing data to ensure its integrity and quality.
- Setting the pre-defined data security and privacy measures in place, including anonymization, de-identification, data minimization, and more.
- Curating and labeling data for training purposes.
- Establishing data governance and maintenance strategies.
Developing or selecting a conversational AI model
Considering the complexity of your conversational AI technology, your development team can either build a custom AI model or leverage a pre-trained one (usually the case with LLMs) and adjust it to the company’s knowledge base and use cases. In healthcare, developers often select or develop models that offer a high degree of explainability.
Training and fine-tuning
Once the developers select or develop a conversational AI model, they calibrate it with the prepped data and fine-tune it for a specific healthcare task. The model’s performance is evaluated against different metrics, including accuracy, precision, recall, and others.
Also, your AI development team takes care of the following:
- Employing targeted fine-tuning techniques to eliminate bias.
- Incorporating user feedback about the model’s accuracy and clarity from early user testing.
- Optimizing the model for real-time interactions.
- Setting up a continuous training and evaluation pipeline to ensure long-term model accuracy.
Integrating the solution with existing health systems
Once the solution is developed and thoroughly tested, it’s integrated with the relevant healthcare systems. Your development team also implements fallback mechanisms to ensure the data flow remains consistent even in the case of a failed request.
Monitoring and optimizing
To make sure your conversational AI solution delivers on its promise and stays accurate down the road, your development team prepares a continuous monitoring and optimization strategy for the post-launch period:
- Regularly monitoring the AI’s performance against pre-defined KPIs.
- Implementing updates and technical improvements.
- Creating continuous feedback loops with users and clinicians.
- Regularly retraining and fine-tuning the model with new, relevant data.
- Looking for changes in data and monitoring for concept drift.
Challenges of implementing healthcare conversational AI solutions
Despite the broader AI adoption imperative, only 18% of healthcare organizations have an AI-ready infrastructure in place. The rest of healthcare providers still struggle with making artificial intelligence, conversational AI included, a part of their tech estate, mainly due to one or a combination of the following roadblocks.
Data privacy and security
Healthcare conversational AI solutions feed on a wealth of heterogeneous healthcare data, including personally identifiable information (PII). Traditionally resistant to innovation, healthcare players may lack governance frameworks to ensure the security, compliance, and privacy of sensitive patient data in conversational AI solutions.
Proactive AI data preparation, along with laying down solid governance structures, will help healthcare organizations gear up for responsible AI adoption. This preparation process includes:
- Data classification and tagging activities to classify data by its sensitivity level
- Data de-identification and anonymization to reduce the risks related to PII
- Encrypted data pipeline development for secure data handling
- Dynamic consent frameworks to ensure patient explicit consent for using their data
- Data governance and AI governance frameworks with operational and technical safeguards
Integration with existing healthcare systems
If organizations aim for AI’s smooth fit with their existing tech ecosystem and healthcare workflows, integration flows must be thought through early in development. Existing legacy systems, intricate clinical workflows, and the lack of standardized data protocols are common barriers to the integration strategy.
To overcome those barriers, AI developers need to align conversational AI solutions with healthcare data standards such as HL7 FHIR and use highly interoperable APIs. Also, mapping the logic of conversational AI solutions to real-world clinical scenarios will not only make the integration technically sound but also functionally and clinically useful.
Accuracy and reliability of AI-driven medical advice
A high-stakes, terminology-rich healthcare data environment demands the utmost accuracy from conversational AI, as inaccurate medical advice can lead to serious consequences. Core to this accuracy is high-fidelity healthcare data, whose accuracy, completeness, consistency, and reliability directly translate into trustworthy artificial intelligence solutions.
However, traditionally fragmented healthcare systems, varying terminologies, and systemic data challenges continue to haunt healthcare organizations. To overcome these gripes, healthcare providers need to define clear standards and protocols for data collection, storage, and management, along with unified medical terminologies and data formats. Automated data validation tools will also prevent faulty data from entering AI workflows and generating unreliable outputs.
Besides data, the architecture of the conversational AI solution should also take into account the complexity of healthcare data. For example, the underlying NLP models must be domain-specific (BioGPT, Med-PaLM, etc.) to take clinical terminology, abbreviations, and context-sensitive language in strides.
💡 Multi-agent architecture is an emerging approach to developing conversational AI solutions in healthcare. Thanks to multiple sub-agents (i.e., LLMs) that run simultaneously, the solution can dynamically choose the most suitable model to achieve a specific goal, which is especially helpful for healthcare-related tasks that require specialized knowledge and high accuracy.
Securing physician buy-in and ensuring patient trust
Both healthcare providers and patients might be wary of artificial intelligence due to its black box nature and the potential for errors stemming from that opaque decision-making process. To break the ice for smoother adoption, healthcare innovators should anchor conversational AI solutions in an evidence-based, explainable, and transparent design.
It means that AI must build on clinically validated guidelines and peer-reviewed research to qualify as trustworthy and keep patient safety at the center of its intelligence. The XAI approach to development that encompasses the selection of inherently transparent models, explanation techniques, and other precautions will also ensure more justifiable outputs.
Regulatory and ethical considerations
The absence of a unified and definitive regulatory rulebook makes healthcare conversational AI a challenging project to pull off. According to existing regulatory U.S. guidelines, healthcare AI applications generally fall into the high-risk category, requiring developers to fulfill quite a few criteria to get the regulatory green light on their AI solutions.
These criteria include detailed documentation on the system and its purpose, the use of high-quality datasets, risk assessment and mitigation systems, and other requirements. AI adopters must also demonstrate the clinical validation for conversational AI systems, explain data provenance, and have human oversight mechanisms in place.
The future of conversational AI in healthcare
Today, most conversational AI solutions are developed with a single use case in mind, whether it’s appointment scheduling or patient routing. In the coming years, we’ll likely see multi-bot ensembles, composed of multiple conversational chatbots, that can handle a range of tasks. United by a single master interface, these solutions will enable empathetic, multi-modal interactions across care, administration, and patient engagement areas, eliminating fragmented user experiences.
If you want to get a head start on your conversational AI solution and future-proof your healthcare offerings, Orangesoft’s expertise in healthcare AI development can help you with that. Since 2011, we’ve been helping healthcare companies flip the script on their approaches with innovative tech solutions. Reach out for a free consultation about your health tech project.