Given the life-critical nature of healthcare, the stress of its staffing shortages, and the pressure of its rising costs, the advancement of AI provides crucial support. According to HIMSS, 86% of healthcare organizations already have some form of AI in play, with AI applications in healthcare spanning administrative and clinical functions.
Drawing from our firsthand experience with AI in healthcare and insights gathered from real-world AI applications presented at global health tech conferences, the Orangesoft team has prepared a list of ten impactful AI solutions already revolutionizing the industry.
AI to automate administrative workflows in healthcare
The admin burden costs the U.S. healthcare industry about 25% of the industry's annual $4+ trillion budget. No wonder healthcare professionals were quick to integrate AI to ease that clinical practice burden. According to statistics, AI is currently most prevalent in administrative tasks, with 36% of healthcare workers leveraging it for transcription and 29% using it for routine communication.
Healthcare administrative tasks that are particularly well-suited for AI automation include:
- Financial transactions — prioritizing claims for follow-up with payers, assigning accurate medical codes, accelerating prior authorization processes, and more.
- Administrative clinical support functions — automating scribe-related tasks, synthesizing care summaries for referrals, optimizing appointment scheduling, streamlining data entries into EHRs, and more.
- Customer and patient services — automating routine phone conversations, benefit confirmations, triage, and personalizing customer touchpoints.
As for specific artificial intelligence technologies, healthcare organizations invest heavily in generative AI to reduce the administrative burden. Over 70% of healthcare organizations are planning to or have already implemented gen AI capabilities to improve clinician productivity and initial issue resolution.
Ambient intelligence, aka ambient AI, is another popular AI offshoot that has already proved its mettle in facilitating real-time scribe-like capabilities. Tapping machine learning for conversations, ambient listening systems can listen silently in the background and transcribe the conversation live during physician-patient encounters.
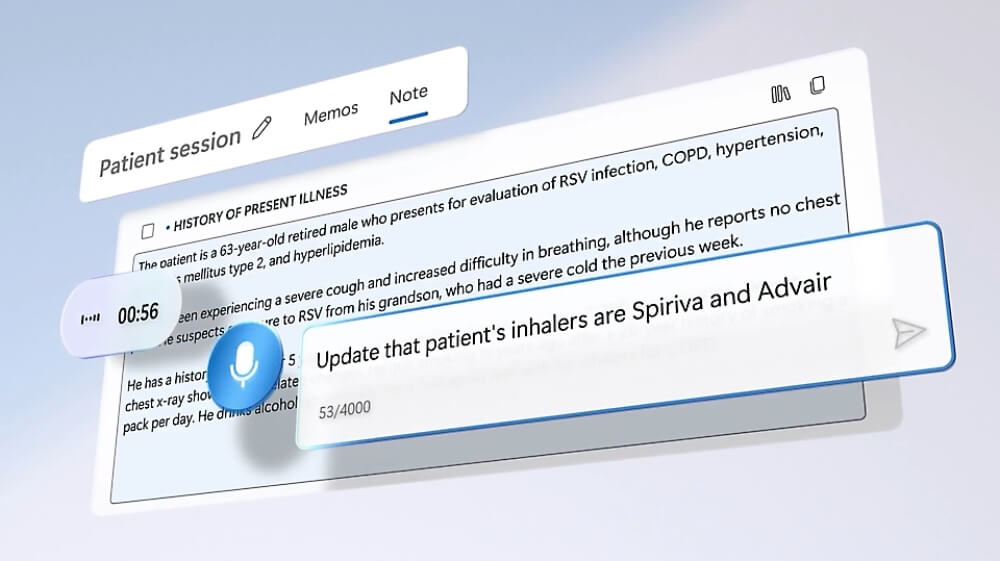
For healthcare providers, ambient AI could also be the gateway to the broader large language model technology. Administratively, it offers quicker and more tangible wins. Data-wise, ambient AI systems do not need extensive data integration to perform listening and transcription tasks.
Building on unified clinical and claims data, voice-activated AI agents are also transforming how healthcare administrative work gets done. With a nuanced context in healthcare operations, they can do the heavy lifting of appointment scheduling, protocol intake, and patient care coordination — and do so autonomously.
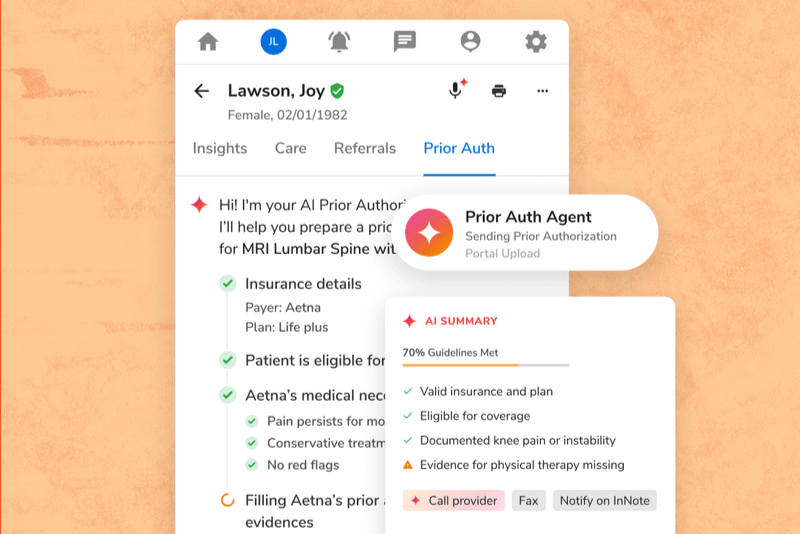
AI to level up medical imaging and diagnostics
Struggling with ongoing imaging backlogs, radiologists experience reporting delays in medical imaging, which can have a detrimental impact on patient outcomes. Globally, up to 68% of practices are looking at unreported radiology examinations.
AI-enabled medical imaging analysis can help radiologists by not only speeding up the interpretation of complex medical images like X-rays, MRIs, and CT scans but also by improving the early medical diagnosis of diseases.
In 2024, over 26% of medical professionals utilized AI systems to analyze X-rays, CT scans, and other medical images. Here's how artificial intelligence can enhance human diagnostic capabilities when it comes to medical imaging:
- Delineating regions of interest within medical images, such as tumors, blood vessels, or cells, for planning targeted interventions and therapy delivery.
- Detecting subtle patterns and abnormalities to spot the onset of diseases such as cancer or cardiovascular conditions that would otherwise go unnoticed.
- Fetching insights related to unique variations in anatomy, physiology, and disease characteristics to inform personalized treatment regimens.
- Reconstructing CT images to limit radiation exposure and improve image quality.
- Delivering augmented visualizations and navigation assistance to surgeons based on combined preoperative and intraoperative imaging data.
With generative AI, radiologists can also spend more time investigating medical imaging, while foundational models can take over documentation tasks.
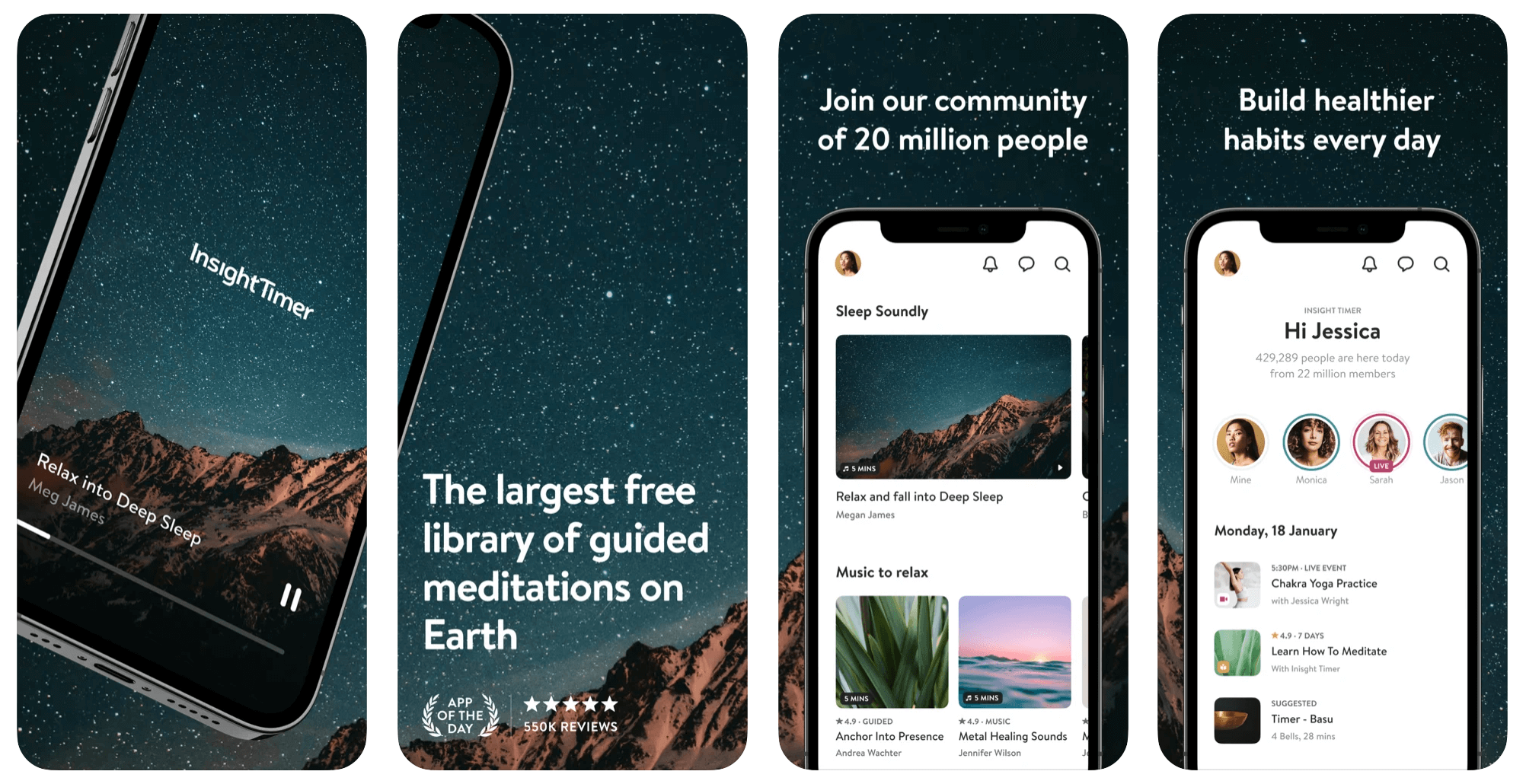
AI to accelerate drug discovery and development
Bringing a new drug to market requires an extensive 10 to 15-year development process that also comes with a multi-billion-dollar price tag. To shorten the drug development lifecycle and optimize costs at every stage, biopharma, and life sciences companies pin their hopes on artificial intelligence.
Proven applications of the AI technology across the biopharma operations include:
- Drug discovery — generating drug molecular structures, identifying potential drug targets, predicting the toxicity of drug candidates, and more.
- Clinical trials — automatically generating artifacts like study protocols, selecting suitable patient subgroups, consolidating and processing clinical trial data, and more.
- Biopharma manufacturing — improving quality control, predicting supply chain disruptions, simulating manufacturing processes for virtual testing, and more.
- Pharmacovigilance — processing ICSRs, breaking down patient narratives, monitoring sentiment on social media and online forums, and more.
And it doesn’t end here: the capabilities of predictive analytics, advanced data modeling, and natural language processing make AI a universal tool in the biopharma sector, which might yield an additional $254 billion in operating profits worldwide by 2030.
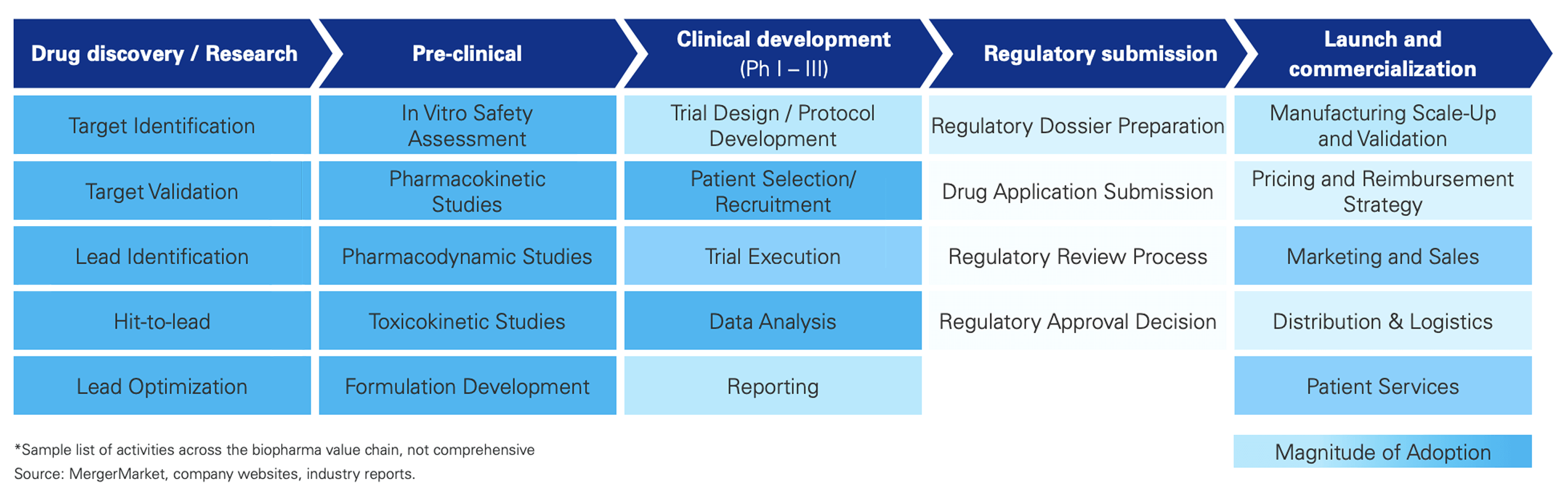
Over the last few years, the biopharma sector has also warmed up to generative AI. When applied to drug research and development, gen AI could shorten the time-to-market by up to 4 years and the costs by up to 45% per successful drug. However, technology adoption within the industry remains in its early stages.
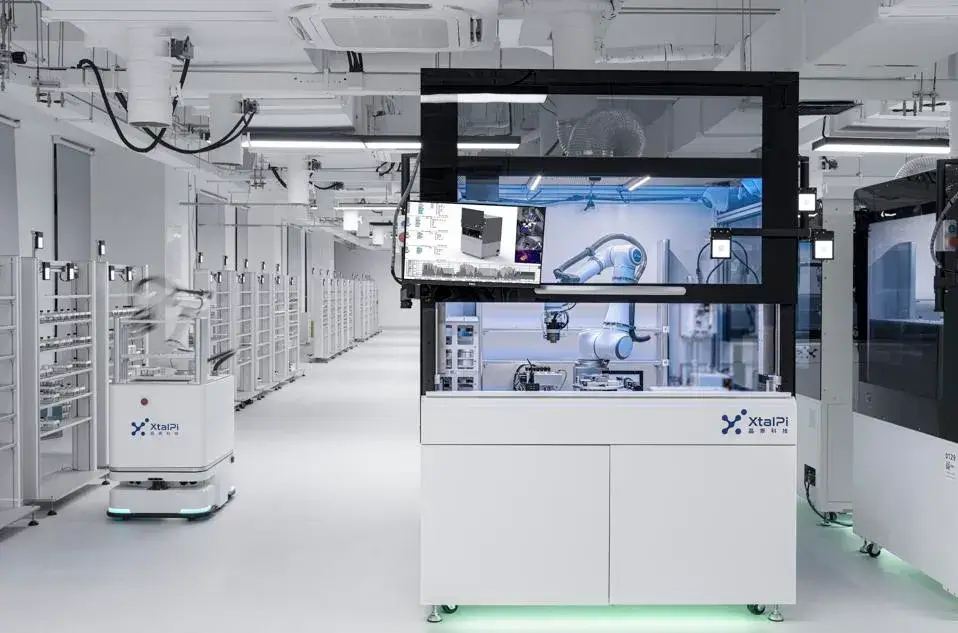
Predictive analytics to prevent diseases
Preventable or treatable diseases account for five of the top 10 leading causes of death in the U.S. Had the focus been on prevention over treatment, the statistics would look different. AI-driven predictive analytics is reorienting the healthcare system towards prevention by providing the tools to run real-time healthcare data analytics on multiple channels — and do so at scale.
In particular, artificial intelligence is actively adopted in such areas as:
- Risk stratification — By analyzing lifestyle, medical history, and social factors, AI algorithms can assign a patient’s risk category — low, medium, or high — allowing for low-effort, at-scale screenings for conditions like cancer, mental health issues, and other diseases.
- Personalized prevention plans — Based on individual risk profiles, ML or generative AI can suggest lifestyle modifications, nutritional interventions, and personalized exercise regimens.
- Population health management — By combining a wide array of data types, including electronic health records, genomic data, environmental variables, and other population-level data, ML models inform targeted public disease prevention initiatives.
- Genomic-based risk assessments — AI consolidates and analyzes clinical risk factors, family history, polygenic risk scores, and monogenic mutations to produce tailored risk assessments based on genomic data.
However, to churn out reliable predictions, AI must be trained on accurate, complete, and representative data and integrated into clinical workflows.
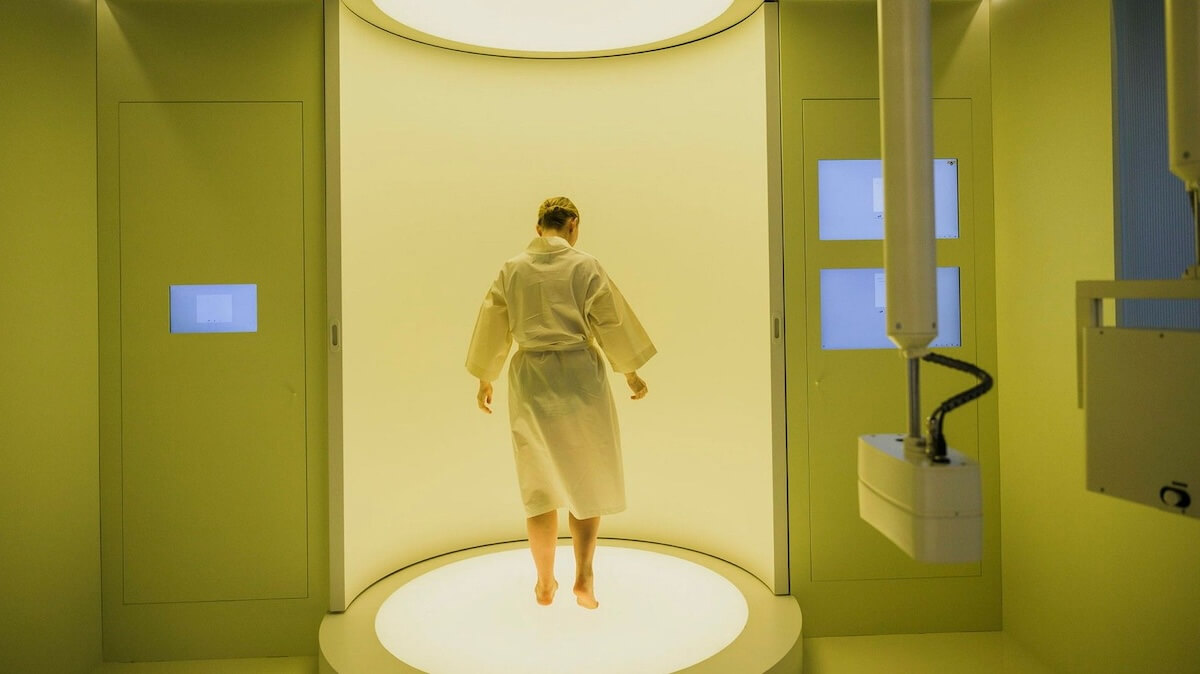
AI-powered chatbots and virtual health assistants to elevate the healthcare experience
According to statistics, consumers are voting with their feet for self-service in health care. 78% of them are extremely interested in confirming their coverage online, while 76% of consumers want to complete pre-visit questionnaires at their own convenience.
Tech-wise, AI-enabled healthcare chatbots are one of the tools that front the movement towards self-service in the healthcare sector. Kitted out with NLP, sentiment analysis, and, if suitable, generative AI, smart chatbots can:
- Serve tailored, conversational post-visit guidance and answer common FAQs by feeding on end-of-visit clinical summary reports and pre-defined guidelines.
- Perform the first round of assessments and offer guidance on appropriate next steps based on patient-reported symptoms and approved triage protocols.
- Automate appointment scheduling and help patients verify their appointments via a conversational interface.
- Help chronic disease patients effectively manage their condition by offering conversational support for chronic disease management based on pre-approved lifestyle and medication tips combined with patient input.
Unlike conversation-focused AI-powered chatbots, virtual health assistants are more workflow-oriented and integration-based. They have more autonomous intelligence and the capability to go beyond a conversational interface to take the capabilities of AI chatbots up an ante:
- Provide personalized recommendations and post-visit guidance based on medical data, EHR data, post-visit follow-up notes, behavioral and lifestyle data, and even patient communication style.
- Enable proactive and predictive triage for remote patients by drilling down into patient-reported symptoms and determining the necessary level of care.
- Facilitate appointment scheduling, dynamically adjusting providers’ schedules, and serving cleared spots to patients.
- Help chronic disease patients effectively manage their condition by offering personalized lifestyle and medication adjustments based on wearable device data, diet logs, and readings.
In 2024, the patient adoption of AI chatbots or voice assistants reached near-universal levels: over 8 in 10 (81%) consumers have turned to such tools to get support from a healthcare provider, with the majority of feedback skewing positive.
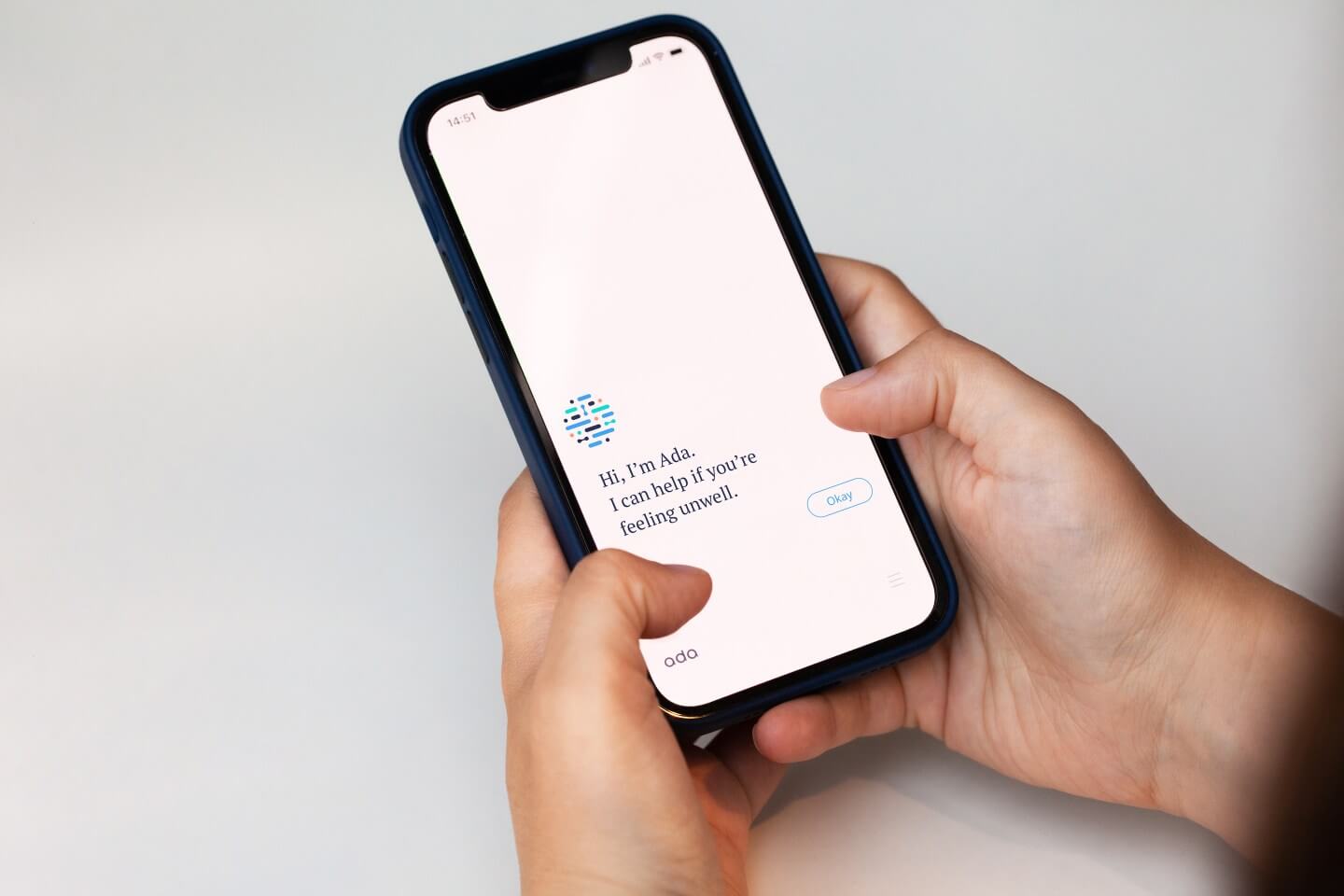
Wearable AI to advance patient safety and supercharge clinical decision-making
Unlike traditional wearable devices, AI-enhanced wearable devices can process multiple types of patient data right on the wearable device or very close to it to almost immediately notify clinical staff or offer context-aware suggestions.
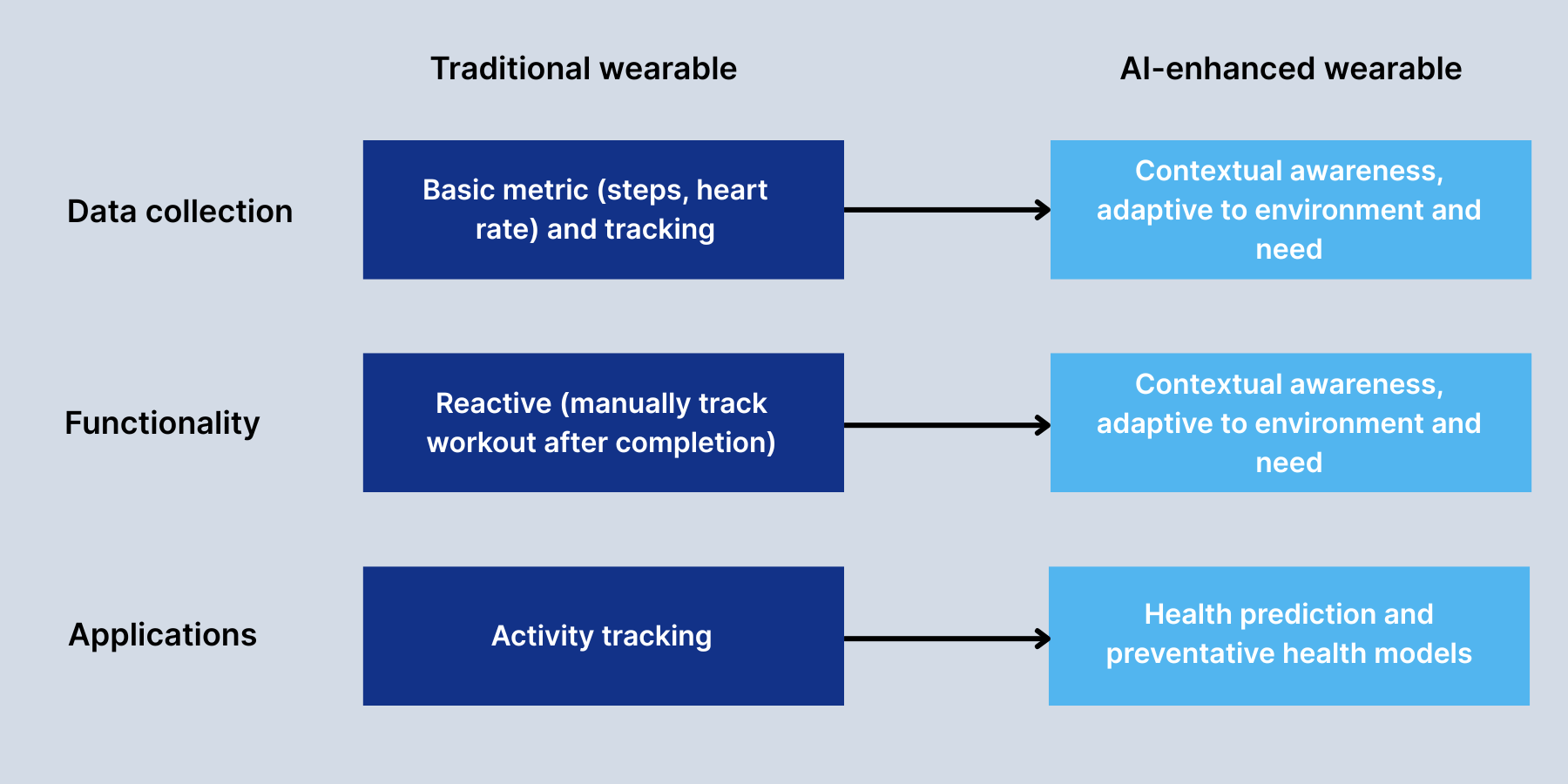
Although this technology is still in its early stages, wearable AI has already proved effective in informing clinical interventions:
- AI-enhanced glucose monitoring systems can both keep track of blood sugar levels and predict critical blood sugar changes well in advance to trigger preemptive interventions.
- Augmented with AI, cardiac monitors can predict cardiac arrhythmia events with high accuracy, enabling earlier detection.
- In areas with limited healthcare resources, AI-powered PPG wearables can predict dengue fever deterioration hours ahead.
Moreover, wearable AI devices can serve as critical safeguards in hectic clinical settings to bolster patient safety protocols:
- In operating rooms and ICUs, wearable AI can determine the contents of syringes and detect medication administration errors.
- In acute care settings, such systems can also classify bleeding lesions in real time, detect early signs of respiratory distress, and alert the staff to post-operative complications.
- Invaluable in fall risk detection, wearable AI devices can track a patient’s movement to identify a patient in the hospital as a fall risk.
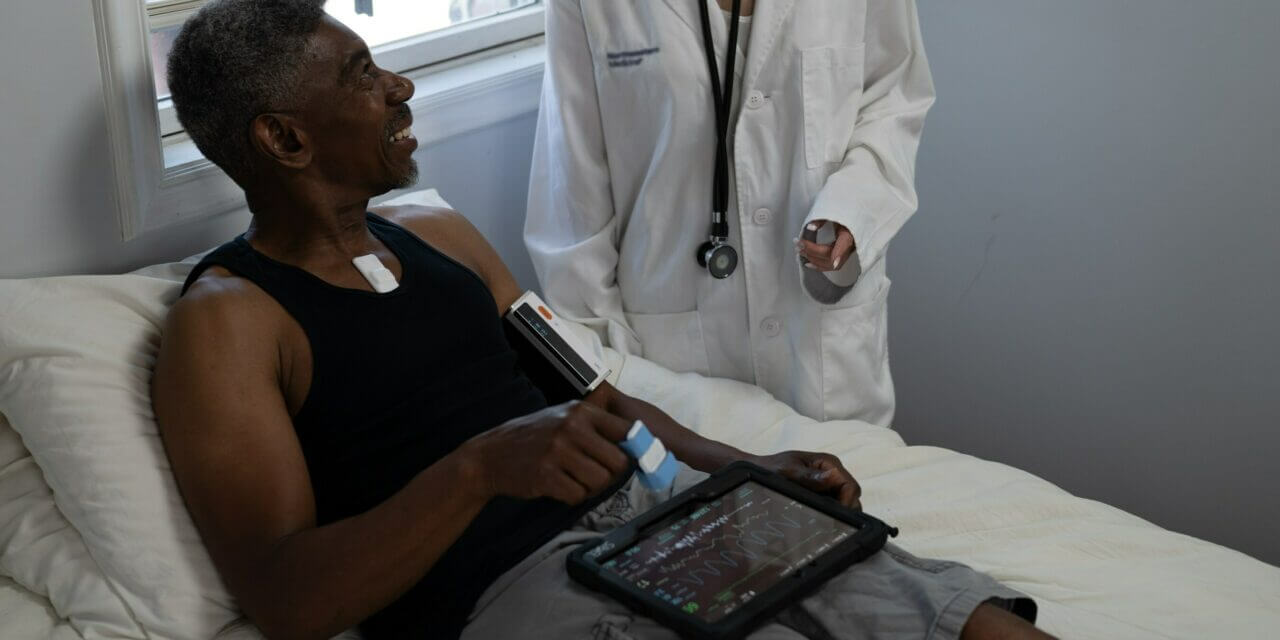
AI collaborative surgical robots to enhance surgeon performance
Traditionally, robotic surgical systems operated according to the ‘master-slave’ dynamic, with the surgeon fully controlling the process. However, with the cutting edge of artificial intelligence in healthcare, robotic surgery got a facelift thanks to greater autonomy and the ability to automate erstwhile manual tasks.
The da Vinci System, a famous robotic surgical system with built-in AI feedback capabilities, reports over 12 million robotic surgery procedures performed with it. Designed to turn surgery from open to laparoscopic, it relies on AI analysis of sensor data to prevent tissue damage, while AI-enhanced 3D images visualize anatomical structures with extreme precision.
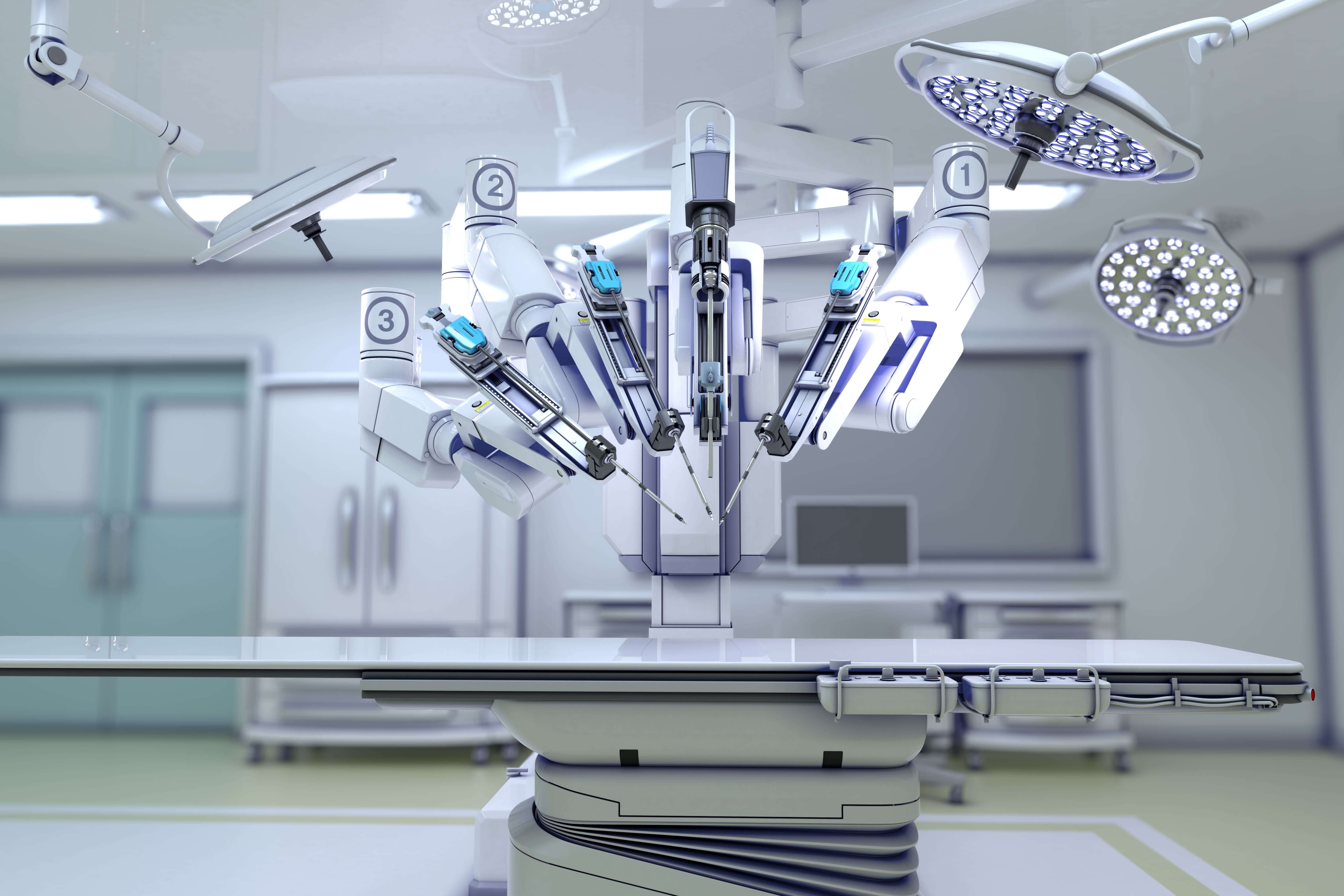
Besides applications featured in the da Vinci Systems, AI in robotic surgery is employed to:
- Peer into surgical field images in real-time to pinpoint critical vascular networks, anatomical structures, and tumor margins.
- Enable motion control and path planning to improve the movement of surgical instruments.
- In combination with haptic feedback, AI can provide surgeons with a better grasp of tissue through the robotic interface.
- Analyze and sum up optimal patterns for surgical procedures to enhance the control accuracy of surgical robots to submillimeter precision.
AI to operationalize remote monitoring data
In 2024, over 50% of healthcare leaders reported using remote patient monitoring systems for chronic disease management. Given high marks for their ability to monitor patients continuously between clinical visits, RPM systems have a few caveats, with data deluge being the chief one.
Artificial intelligence capabilities can help care teams wrestle with the avalanche of RPM data and prioritize valuable insights over extraneous ones. In particular, AI algorithms bode well for the following RPM use cases:
- Processing health data in real-time to dissect fine patterns and deviations from baseline health data and to spot early warning signs of clinical deterioration.
- Informing therapy or medication adjustments based on changing patterns in patient health data.
- Putting together a unified profile of the patient’s health based on input from diverse sources while also normalizing and deduplicating it.
- Sending alerts to healthcare providers when critical thresholds are reached or when there is a likelihood of adverse health events (readmissions or complications) according to historical data tendencies.
- Integrating remote monitoring data into EHRs to improve clinical workflows.
At the core of all applications is the AI’s ability to filter out statistical noise, distill relevant features, and contextualize data with patient history and clinical guidelines. Not only do these enhancements improve the signal-to-noise ratio in RPM data, but they also enable proactive and personalized care for remote patients.
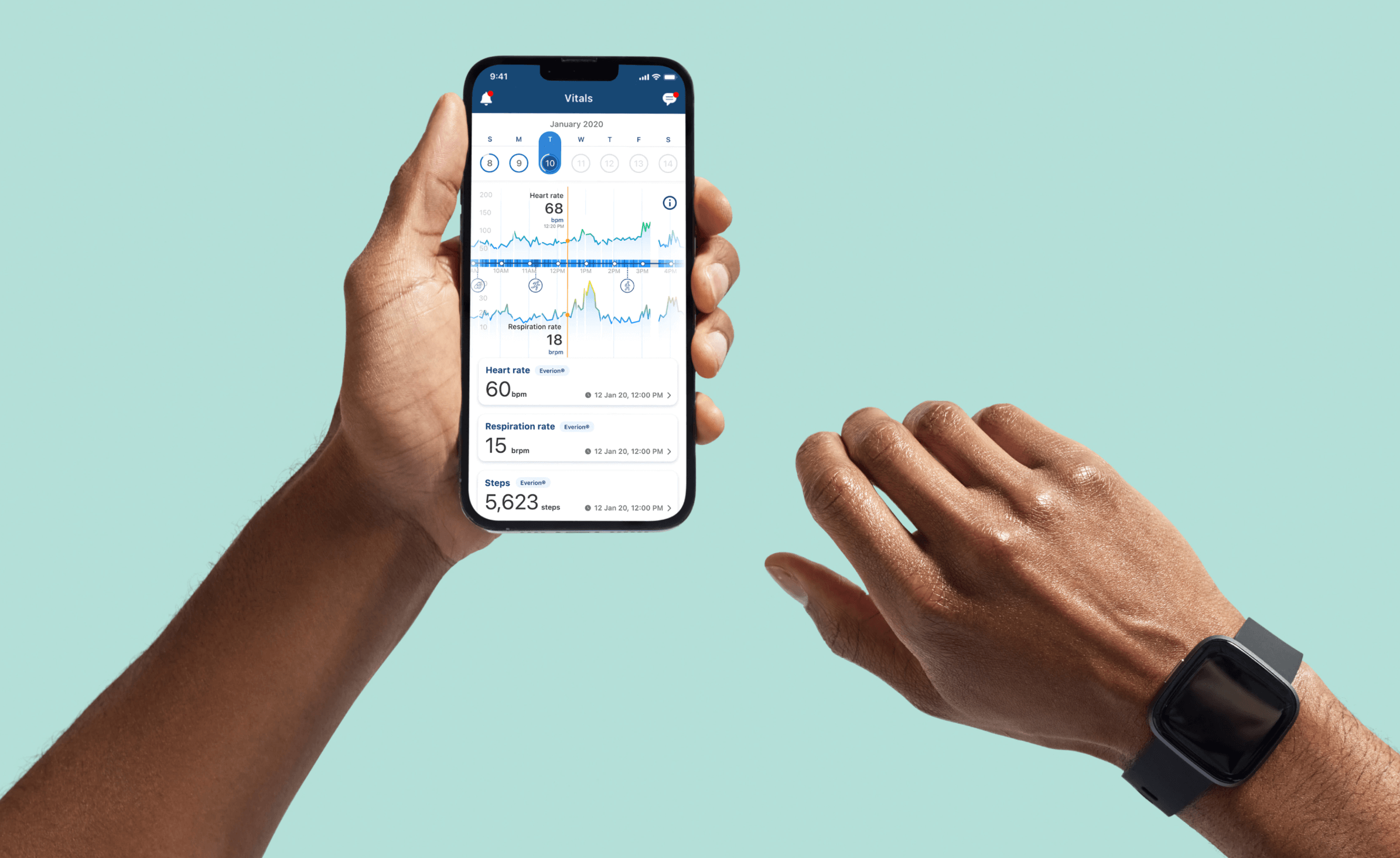
AI to transform elder care
95% of U.S. adults 55+ prefer to age safely within their home environment. However, demand exceeds supply: the US expects a 25% increase in home health and personal care aide needs by 2031, which exceeds the current system's capacity.
Coming to bridge the gap and help seniors age healthier and happier, artificial intelligence pitches in with the following applications:
- Fuelled by AI, voice-operated smart assistants empower seniors to track medications, stay connected with their loved ones, improve their well-being with meditation sessions, and engage in cognitive stimulation with games.
- Device-based AI systems can supplement RPM setups by monitoring and analyzing sounds within a home environment to alert care teams to falls and emergencies, unusual activity levels, or environmental hazards.
- In senior living communities, fully ambient AI systems use AI-powered video analysis to deliver insights on fall patterns and resident behaviors to improve care coordination between staff members.
- By analyzing health, demographic, family, and existing coverage data, AI-enabled solutions can deliver personalized care forecasts and cost estimates for long-term care to give seniors and their families financial clarity and peace of mind.
However, with 59% of older adults thinking that technology is not designed with their age in mind, innovators have to put age-friendly design at the core of their AI solutions to make sure the technology reaches the target audience.
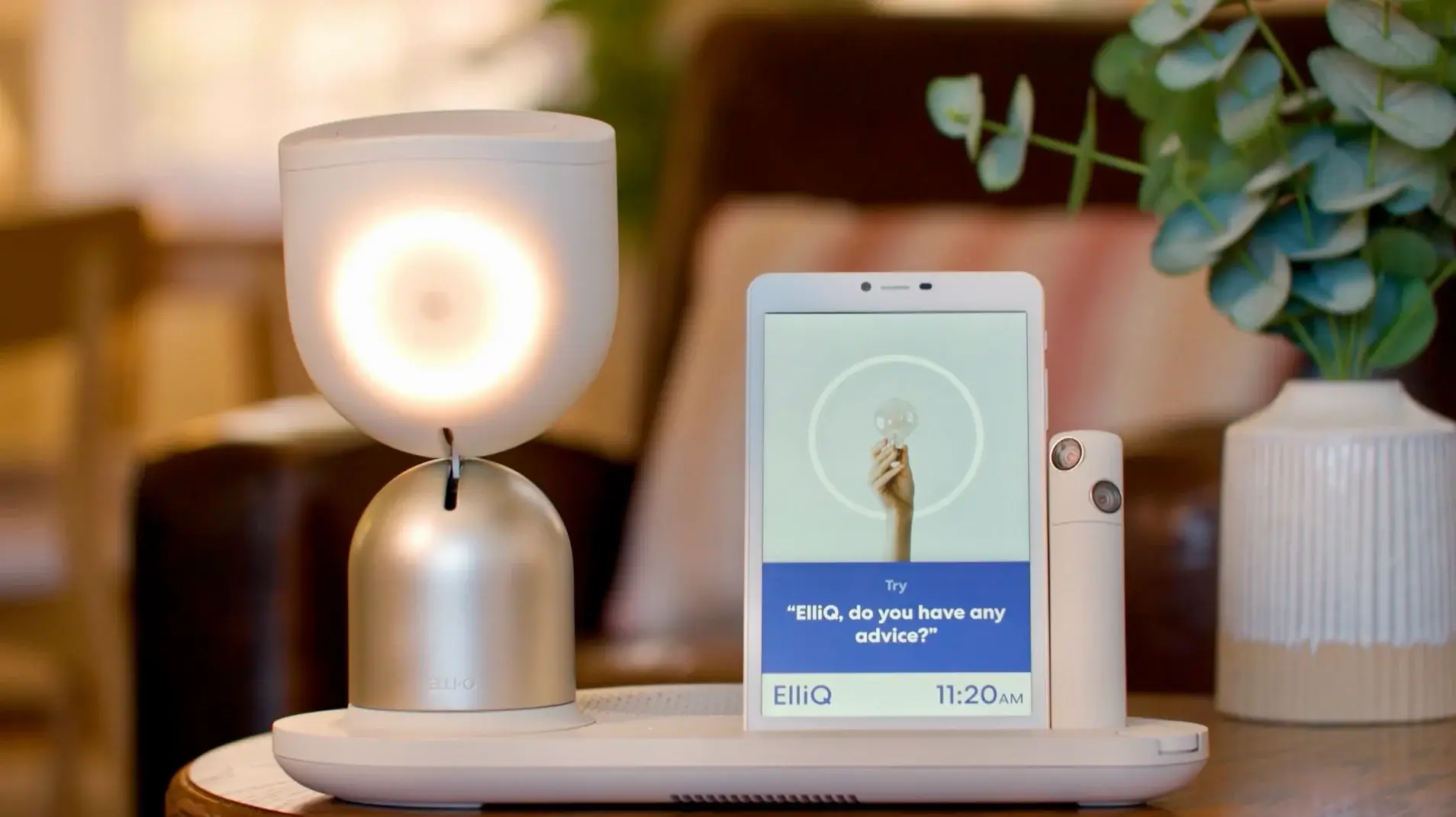
AI to expedite and enhance mental health diagnosis and therapy
Currently, around 76-85% of severe mental disorders go untreated in low and middle-income countries compared to 35-50% in higher-income nations. The reasons for such stark numbers vary, with high costs of mental healthcare and associated stigma prevailing.
Artificial intelligence can improve the accessibility of mental healthcare and give mental health professionals the tools to objectivize the DSM-5 framework and better handle the treatment side of things.
In mental health, the leading AI applications include:
- Risk assessment — by analyzing an individual’s profile, genetic risk, and environmental factors, AI can evaluate the risk of a specific mental health disease for a given patient.
- Detection of mental health disorders at prodromal stages — AI algorithms can sift through clinical notes, electronic health records, diagnostic tests, and behavioral patterns to accurately predict a disease. Equipped with face recognition and NLP, AI algorithms can factor in speech patterns and facial expressions to calculate the likelihood.
- Personalized mental health therapy — AI-powered chatbots and virtual therapists analyze self-reported data, data from platform interactions, and physiological data to deliver tailored CBT, talk therapy, and other evidence-based practices.
- Mental health self-management — AI mental health apps, often paired with a built-in chatbot, provide the tools for individuals to track emotional health in real time, develop coping skills, get tailored self-care tips, and navigate their recovery journey.
- Remote monitoring and crisis intervention — multimodal AI solutions can identify individuals at risk of suicide by spotting warning signs in social media posts, speech activity, textual communication, behavioral data, and more. If programmed, such solutions can quickly send clinicians a notification about at-risk behaviors.
Wearable neurotech, the latest gizmo in mental health innovation, also relies on AI for adaptive feedback or personalized neuromodulation. Wearable neurotech devices apply brain stimulation from outside the body, with AI identifying the optimal location, intensity, and frequency of magnetic pulses based on brainwave data.
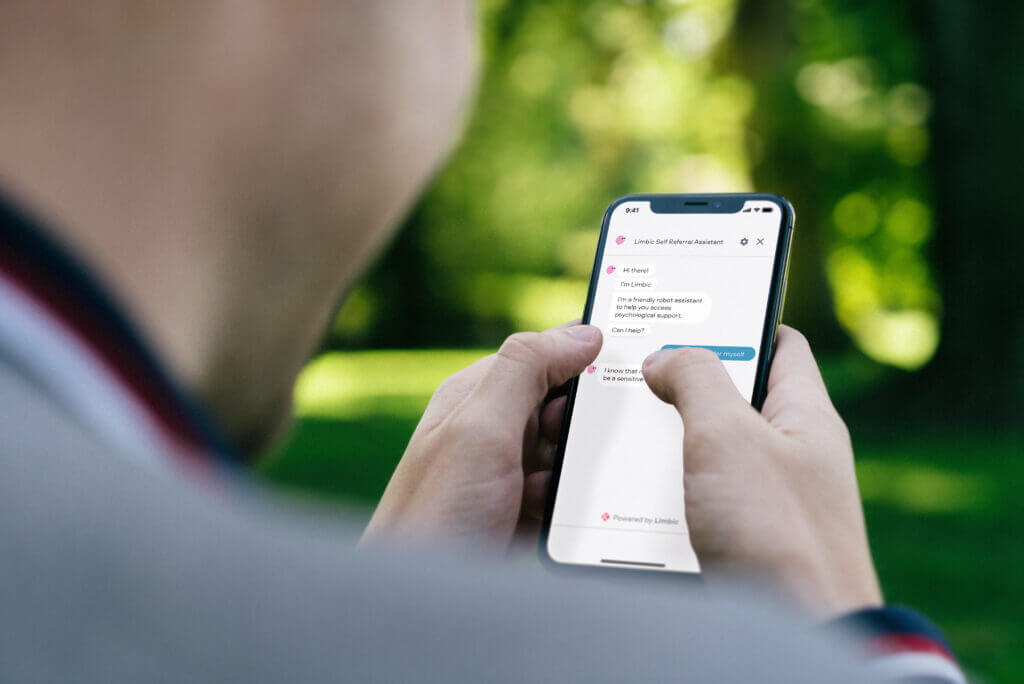
How to bring AI into healthcare safely and with maximum impact
Like any promising technology, artificial intelligence has a lot of duality to it. Its immense automation potential and unmatched analytics chops are counterbalanced by significant ethical quandaries and a complex set of other challenges. Therefore, healthcare requires a security-first framework for AI implementation to ensure benefits outweigh potential risks.
Start with high-value use cases with easily quantifiable impact
Contrary to an AI-first strategy, a measured use-case-driven approach to healthcare AI makes a better mesh for the industry as it focuses on high-impact solutions that can then easily be scaled across the organization. According to this approach, healthcare providers need to select one or a few use cases with the highest feasibility for AI implementation, lowest risks, and minimum data and technical gaps.
Developing initial proof of concepts for such use cases should serve as a foundation, allowing organizations to create AI momentum, gradually build the infrastructure, obtain concrete evidence of the technology's value, and ease employees or patients into fully adopting the technology. Depending on the data availability and tech infrastructure, examples of high-impact use cases may include:
- Readmission prediction (directly translates to cost savings, AI could be trained on existing EHR data)
- Chatbots for appointment scheduling (directly translates to reductions in no-show rates; scheduling data is typically well-structured)
- AI-driven medication adherence monitoring (low-risk, medication prescription, and adherence data are often accessible in EHRs and pharmacy systems), and more.
Determine the data scope and tech capabilities
To develop a reliable and accurate AI model, organizations must train it on broad, high-quality, and well-labeled data, which is often scattered across different healthcare systems. So, before getting started on healthcare AI, companies or their development team assess the data landscape, identify the data needed for training and its availability, and prepare the data for development.
Warm-up data activities also include data integration, preprocessing, labeling, and annotation. Data preparation activities must be accompanied by data governance and security strategies to minimize the risk of AI implementation and make sure your AI solution doesn’t perpetuate biases. These strategies usually define data access controls, de-identification techniques, and anonymization methods, explicit policies to manage PII and PHI, and more.
Along with data readiness, healthcare innovators need to evaluate the existing technical infrastructure, including the apps, models, APIs, and other tools, to see whether some of their tech abilities can be reused for AI projects and understand the necessary tech investments.
Design a comprehensive AI governance framework
Effective AI governance is what draws the line between responsible innovation and uncontrolled, potentially harmful deployment. It outlines how the organization will use AI and provides a structured approach to risk management and ethical considerations of AI in healthcare.
Key elements of an AI governance framework in healthcare include ethical guardrails such as bias prevention techniques, accountability and responsibility mechanisms, data security methods, capabilities for continuous monitoring and evaluation of AI performance, and other critical components.
Lead with an agile, iterative approach
An iterative test-and-learn approach aligns with the dynamic nature of artificial intelligence, allowing companies to minimize risks and optimize their development costs. A salient part of this approach is systematic testing that helps AI engineers pinpoint an AI model best suited to the specific use case.
Iterative development cycles, rapid prototyping, and continuous feedback from clinicians and patients, along with real-world data validation, not only accelerate AI innovation but also build trust among stakeholders by demonstrating transparency, reliability, and a commitment to ethical AI practices.
From strategy to implementation with Orangesoft
Every AI healthcare project involves a complex interplay of compliance requirements, security challenges, and development considerations. Since 2011, Orangesoft has been helping healthcare organizations and health tech companies safely and effectively implement the latest technologies, including AI.
With hands-on experience in healthcare regulations such as HIPAA, FDA, GDPR, and other regional laws, Orangesoft develops custom AI solutions that seamlessly integrate with existing healthcare systems and data sources. Contact our team to assess the feasibility of AI for your specific use case, get a strategic roadmap for your next AI project, or develop a smart solution from scratch.